All your sports betting on your hands – international sports such as football, basketball, and even your local sports in Bangladesh. There are, if you’re into esports or other more niche options. The wide range of choices means that there’s something for everyone — from betting on mainstream leagues to niche competitions.
How to Place a Bet on Sports?
How about placing a bet on sports? All you need to do is login, pick your ideal event and sport to bet on, your odds level, and place your bet. If you’re feeling bold, you can place a live bet while the game is still going! All very intuitive and be sure to check out an betting bonuses you find while placing your bets!
Other Casino Games
Not feeling the slots or live games? No worries! R777 also has a variety of other casino games to keep the excitement going. Resource offers everything from fishing games and bingo to cockfighting. These games are alternative, but equally (or even more) fun and rewarding.
Some of the most popular games in this category include:
Fishing Games: These games will give you special content, unlike other types, you can catch not only fish but also big money!
Bingo: Timeless, fast-paced, always thrilling.
Cockfighting: The little more power game.
These are distinctive games that are ideal for mixing things up a little, bringing an extra element to your experience in the casino.
Bonuses and Promotions
Who doesn’t love free spins, deposit matches, goods only for rewards? R777 Casino bonuses are far from a marketing ploy—they represent an opportunity for you to increase your winnings, play for longer, and add excitement to every bet. Whether you’re a newbie in search of a friendly boost or a seasoned bettor pursuing VIP perks, etc. Now, we explore the bonuses and promotions that placed this casino in the spotlight.
Welcome Bonus and Steps to Claim It
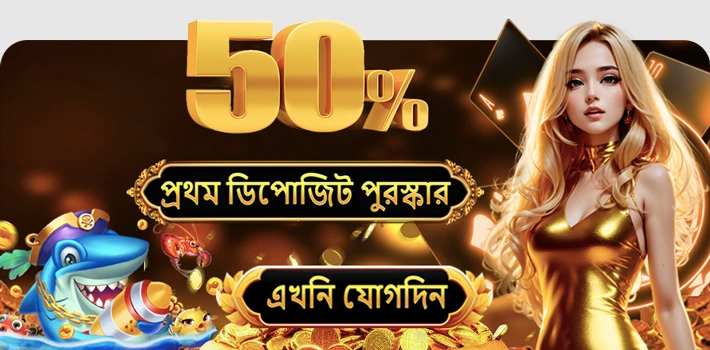
Walking into a casino should be like walking into a party, no? This is why R777 Casino welcomes every new player with a huge welcome bonus. If you’re new to signing up, expect a healthy deposit match that’s a multiple of your first deposit so you have more on hand to try the platform.
So, how do you claim it? It’s simple:
- Sign up for a new account — it only takes a few minutes.
- Log in and make your initial deposit (see minimums to qualify).
- Use your promocode (if any) to activate your bonus.
- Get to see your bonus funds land in your account and if you want to play slots, live games and sports betting right now!
The welcome bonus most often also includes freespins for the best slot machines where you can get started and then you will risk as little of your own money as possible. And if you’re the type who loves to go high stakes crazy with Aviator or Crash, this boosted bankroll can allow you to go bigger for a chance to win even bigger.
Loyalty and VIP Programs
Rewards range from the table to the casino floor, and the establishment ensures that if you stay and play, you are valued. Each dedicated & loyal player gets the benefits of the VIP and loyalty programs come into play where they get exclusive perks, increased betting limits, and even personalized promotions.
It’s sort of like a video game — the more you play, the more awesome your rewards become. Different VIP levels grant access to cashback bonuses, increased withdrawal limits, early access to new game releases, and invitations to exclusive live tournaments.
High rollers, on the other hand, get a tailored treatment, including personalized deposit bonuses, dedicated support, and gifts. Whens it comes to slot machines, live games or sports betting, the loyalty program makes sure that every spin, bet and wager goes towards something greater.
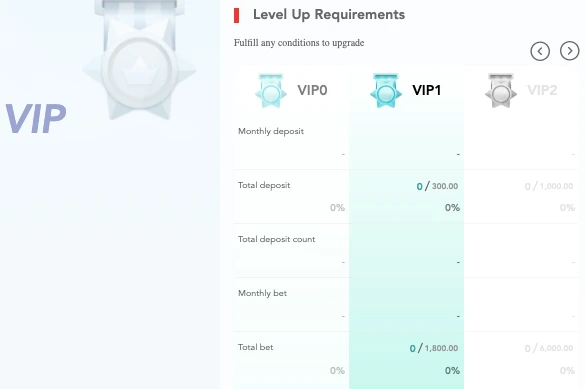
Daily and Weekly R777 casino Promotions
So why not treat yourself to rewards a little early? R777 Casino is a new and exciting online casino where every day brings a new opportunity to claim an additional incentive. From a weekly cashback offer to deposit boosts or surprise freespins, there’s always something on offer.
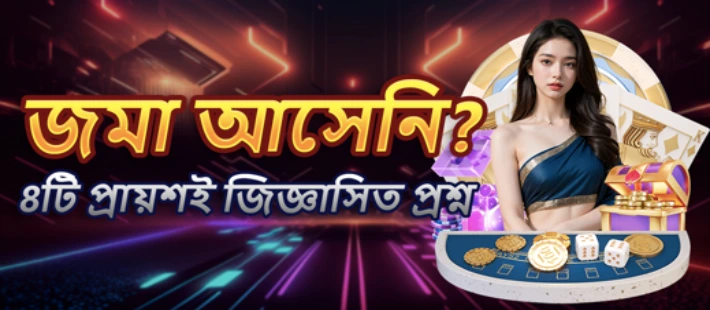
If you are a sports fan there are many different sports betting promotions for major events, often giving you the chance to place a bet with enhanced odds or without any risk. And if you prefer casino games, you may find yourself with reload bonuses, free tournament entries, or exclusive live game rewards.
And we’re not even talking happy hour promos and mystery bonuses, in which you can log in at just the right time to find bonus credits, spins or invites to private competitions waiting for you.
Stay tuned to the promotions page and turn on app notifications to never miss an opportunity to build your bankroll.
Wagering Requirements Explained
Now, let’s get to the fine print. Wagering requirements are associated with every casino bonus, and knowing all about them is the only way to deposit back some money after winning — without surprises.
So what exactly do “wagering requirement” mean? In the simplest of terms, it’s how many times you have to play through your bonus cash before you can take it out. So, if you receive a 100% deposit bonus with a 25x wagering requirement, you must play through the bonus amount 25 times before you can cash out any winnings.
Here’s a quick primer on how various bonuses usually operate:
Bonus Type |
Typical Wagering Requirement |
Games that Count Toward Wagering |
Welcome Bonus |
20x – 40x |
Slots (100%), live games (10-50%), sports betting (varies) |
Free Spins |
15x – 30x |
Slot machines only |
Reload Bonus |
20x – 35x |
Slots, live games, and some sports bets |
Cashback Offers |
None – some have 1x–5x |
Direct credit to balance, minimal restrictions |
Is your passion for slots because if so you are in luck as most slots contribute 100% towards wagering requirements. But if you prefer live games or sports betting, you will have to check which ones are counted (often on a smaller percentage).
The key takeaway? Bonus TermsAlways read the bonus terms before claiming. With good planning, you can convert a bonus into cash you can withdraw.
R777 Casino – Deposits and Withdrawals
More than the games, the bets, or even the bonuses, one of the most important aspects of playing at an online casino is the understanding that your deposits and withdrawals can be managed smoothly and securely. Whether you are making a deposit or withdrawing your winnings, the process is straightforward and easy. Here’s what you need to know when funding your account and cashing your big win.
Available Payment Methods
No one wants to waste time dealing with complicated transactions. That’s why R777 Casino offers a wide range of payment methods to ensure that every player—whether local or international—can deposit and withdraw with ease.
Here are the main options available for Bengali players and beyond:
Payment Method |
Type |
Available for Deposits |
Available for Withdrawals |
Processing Time |
Credit/Debit Cards |
Visa, Mastercard |
✅ Yes |
❌ No |
Instant deposit |
E-Wallets |
Skrill, Neteller |
✅ Yes |
✅ Yes |
Instant for deposits, up to 24 hours for withdrawals |
Mobile Payments |
bKash, Nagad, Rocket |
✅ Yes |
✅ Yes |
Instant for deposits, up to 24 hours for withdrawals |
Cryptocurrency |
Bitcoin, Ethereum |
✅ Yes |
✅ Yes |
Instant for both deposits and withdrawals |
Bank Transfer |
Local and International Banks |
✅ Yes |
✅ Yes |
1-3 business days |
Prepaid Cards |
Paysafecard |
✅ Yes |
❌ No |
Instant deposit |
All payment methods come with secure transactions, meaning you can enjoy your favorite slot machines, sports betting, or live games without worrying about your funds. Whether you want to stick with old-fashioned ways or you’d like to get into digital with crypto, there’s a solution for you.
Minimum and Maximum Deposit Limits
At R777 Casino, the deposit-making process is tailored to suit your betting style like a glove. Whether you’re a casual player only dipping your toes in the water, or a high roller shooting for a bonkers big win, the site caters to budgets of all needs.
Minimum deposit: You can deposit more than ৳500 using most payment methods, but with mobile payments such as bKash and Nagad, this amount can already be ৳200.
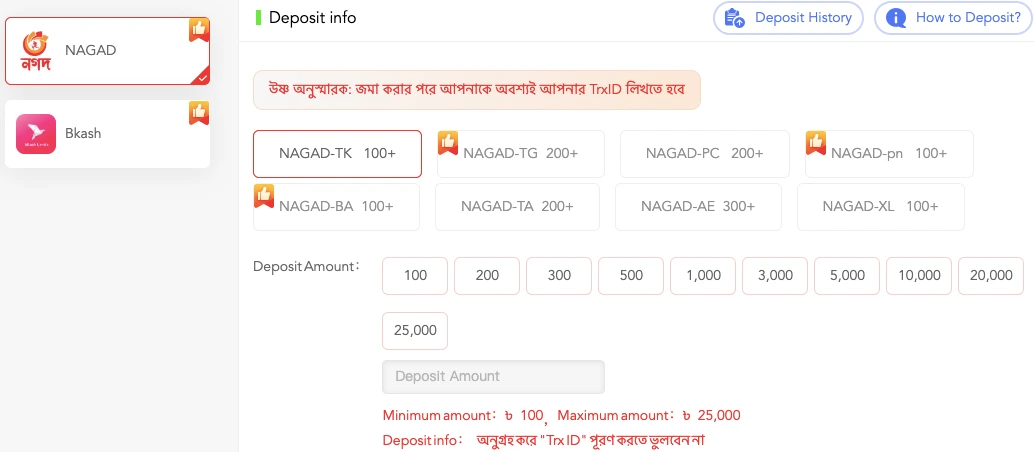
Upper platform deposit: For the brave, you can deposit up to ৳50,000 in one go, with higher ceilings for VIP players.
Also, keep in mind that a lot of bonuses and freespins are related to how much you deposit. Most promocodes need a minimum deposit, so always check the details before making a transaction.
How to Withdraw Your Winnings?
So, you’ve placed your bets, hit a crazy winning streak, and now it’s time to cash out. Withdrawing your winnings at R777 Casino is straightforward, and there are no hidden tricks or delays.
Here’s what you need to do:
- Login to your R777 Casino account.
- Head to the Cashier section and select Withdraw Funds.
- Choose your preferred withdrawal method (e-wallet, bank transfer, mobile payment, or cryptocurrency).
- Enter the amount you wish to withdraw and confirm the transaction.
- Wait for processing, and once approved, your funds will be on their way!
Most withdrawals are processed within 24 hours, but bank transfers may take up to 3 business days. For the fastest cashouts, e-wallets and crypto payments are your best bet.
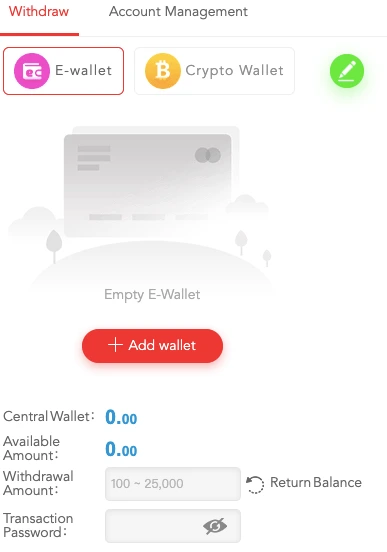
To avoid any issues, make sure you’ve verified your account by submitting the necessary documents. This is a standard procedure for security purposes and ensures that your big win reaches the right person—you!
R777 casino Withdrawal Processing – Time and Fees
Everyone loves a fast payout, and R777 Casino makes sure you get your money quickly. Here’s what you need to know about withdrawal times and possible fees:
Payment Method |
Processing Time |
Fees |
E-Wallets (Skrill, Neteller) |
0-24 hours |
No fees |
Mobile Payments (bKash, Nagad) |
0-24 hours |
No fees |
Cryptocurrency (Bitcoin, Ethereum) |
0-12 hours |
No fees |
Bank Transfer (Local Banks) |
1-3 business days |
May apply based on the bank |
Prepaid Cards & Credit Cards |
Not available for withdrawals |
N/A |
Most payment methods don’t charge any withdrawal fees at R777 Casino, although some banks and third-party services do charge small transaction fees which may be applied depending on the location of the transfer (i.e., international transfer creates a fee). If you’re uncertain, ask your payment provider.
Customer Support and User Experience at R777 Casino
Playing at an online casino should be a seamless process with no hassle, but what if there’s an issue? Perhaps you can’t log in, your bonus didn’t turn on, or you have a question about a withdraw. Whatever the case may be, players can count on customer support to resolve any issue as quickly as possible. While thrilling games, betting, and winnings are key, a premier casino is more about players getting the support they need without a hitch.
How to Contact Customer Support?
Need help? The R777 Casino support team is easily reachable. Whether you have a simple question or have a concern that requires immediate attention, you can get in touch via a range of contact methods.
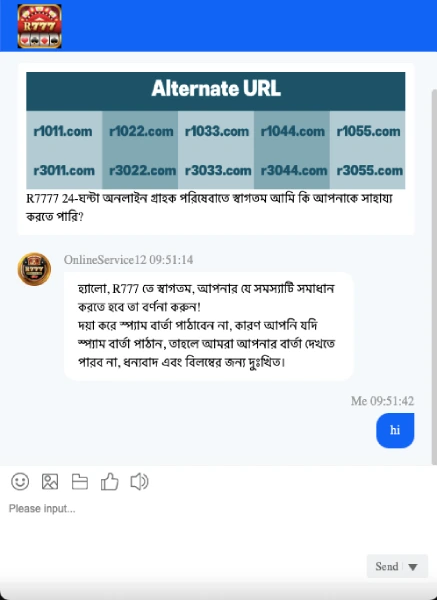
Live chat is the quickest option for assistance. Open all day, every day and calling through will only take a few minutes, connecting you directly with a customer service representative who will be able to help with everything from betting queries to technical issues. If you prefer extensive answers, you can contact their support team, and this is suitable for account verification, bonus-related queries or complicated questions.
R777 Casino caters as well to players who prefer instant solutions by providing a FAQ page that addresses several common questions including those about registration, deposits, withdrawals and bonuses. FelineFriends Sometimes, the answer you seek is a few clicks away.
Common Issues and Troubleshooting
Even the best casinos can have a few hiccups now and then, but the good thing is that most are easy to fix.
Many players get a common problem which is a login issue. If you cannot get into your account, ensure you have typed in the proper username and password. If that fails, reset your password or where applicable, reach out to help desk. If a deposit isn’t showing up in your balance, also check back in a few minutes — sometimes it takes a little time for payment processing.
Bonus activation is another common question asked. If you’ve qualified for a deposit but don’t receive your freespins or betting bonus, this might be due to failing to enter the appropriate promocode or reaching the minimum deposit amount. If you are still having issues the support team can also manually add the bonus for you.
If you have problems with the game itself, for example one of the slots freezes or there is a crash in Aviator, refreshing the page or switching to another device typically solves the problem. Should it still occur customer service is always there to investigate.
User Reviews and Ratings
A casino’s reputation is built on real player experiences, and R777 Casino has received a mix of feedback from players who enjoy its games, bonuses, and fast payouts. Here’s what users have to say about their time:
Great Selection of Games and Fast Withdrawals
⭐ 9/10
“I’ve been playing at R777 Casino for a few months now, and the variety of games is amazing! I love the slots, but my favorite is Aviator—it’s crazy fun and gives you a real chance for a big win. Withdrawals through bKash are super fast, and I usually get my money within a few hours. Highly recommend!”
— Rahman H., Dhaka, Bangladesh
Good Sports Betting, But Verification Took Time
⭐ 8.5/10
“I joined principally for sports wagering, and I need to say, the chances are truly aggressive. The live wagering highlight is smooth, and I’ve won a couple of good wagers on cricket matches. The main explanation I’m giving 8.5/10 is that my most memorable withdrawal took a piece longer because of check, however from that point forward, everything was quick.”
— Imran K., Chittagong, Bangladesh
Best R777 Casino App for Bengali Players
⭐ 9.5/10
“What I love about R777 bet is that it really functions admirably for Bengali players. The application is not difficult to utilize, stores through Nagad are moment, and the rewards are marvelous. The free twists advancements are perfect for openings, and I’ve had a few decent wins! Certainly my go-to online club.”
— Sadia M., Sylhet, Bangladesh
Fun Games, But More Promotions Would Be Nice
⭐ 7.5/10
“I like playing here in light of the fact that the games are energizing, particularly Crash and the live gambling club tables. I’ve won a couple of times, and withdrawals were smooth. My main protest is that I wish there were more advancements for existing players, not simply welcome rewards. Other than that, it’s a strong site.”
— Tariq A., Khulna, Bangladesh
Overall Rating: ⭐ 8.6/10
With a strong game selection, fast payments, and a user-friendly R777 app, this is a favorite among Bengali players. While most users appreciate the casino’s features, a few mention minor delays in verification and the need for more ongoing promotions. Overall, it’s a trusted and enjoyable platform for betting, slots, and live games.
Responsible Gambling and Fair Play at R777 Casino
Gambling must always be fun. If you’re spinning slots, betting on your favourite sports team or chasing a big win in Aviator or Crash, the thrill of the games is what keeps it fun. But as with anything pleasurable, moderation is key. Responsible gambling is a priority, not just a policy at R777 Casino. So let’s discuss how the platform provides fair play, player protection, and responsible betting as for you to enjoy your experience without the need of worrying.
Gambling Regulation & Player Protection
Ever heard the saying “The house always wins”? Well, at R777 Casino, making sure that every player gets a fair shot is priority number one, and it requires some serious magic. The platform has policies for gambling that encourage and promote transparency as well as reasonable play and security.
But first, player protection. When you sign up and play, R777 Casino takes care of your personal data, deposits, and withdrawals. All transactions are encrypted — the sort of thing you would see in high-end financial institutions. So, when you transfer money or receive a bonus, you can rest assured that you are in good hands.
R777 Casino promotes responsible betting habits, beyond the security. No underage gambling, no false promocodes, and no unjust game mechanics. No matter whether you are playing live casino games, sports betting or slot machines, everything is monitored in order to keep the experience safe, fair and fun.
Self-Exclusion and Limits on Betting
Once in a while, a game becomes a bit too exciting. Perhaps you were on a winning streak and want to ride the wave, or maybe you’re chasing loss in a hope to make a comeback. It happens. But here’s the thing — as critical as knowing when to make a bet is knowing when not to.
This is why self-exclusion and betting limits are available at R777 Casino. You can also set limits on how much you deposit, bet, or play, daily, weekly or monthly. If you think you need a rest, you can self-exclude — you won’t be able to log on, make bets or even download the app for a specified period of time.
It isn’t just a feature for people who think they’re betting too much — it’s for everybody to help ensure that gambling never up-ends your normal life. No matter if you are doing it just for the fun of it or if you want to try to score that big win, creating limitations prevents gambling from being a stressful pastime, armeed with it still being a fun endeavor.
Ensuring Fair Play and Game Integrity
Have you ever asked yourself if online casino games are rigged? It’s a reasonable question — as you want to know, after all, when you place a bet that the game isn’t rigged against you. R777 Casino offers a fair playing ground, not a promise, but a system.
All the games, from slots to sports betting, run on random number generators (RNGs). That means, every spin, every card, every bet outcome, is totally random and fair. The casino doesn’t dictate the outcomes — luck and strategy do.
Even live casino games, with real dealers shuffling cards or spinning the roulette wheel, are closely watched to prevent cheating. Every game played delivers an honest experience, thanks to independent audits.
So, no matter if you’re going for broke on spinning machines, risking real chips on live blackjack or making use of your freespins to tempt fate, you can rest assured that the odds are just what they should be – even Stevens.
Final Thoughts
R777 Casino: The New Dimension of Gaming is not just a gambling platform; it is an entire gaming experience that caters to players looking for excitement, variety, and fair play. From slick slot spinning to sporting betting and live dealer experience, whatever you fancy, you will find it here! Every single bet feels like a winning one thanks to generous bonuses, freespins and promotions. With just a few clicks, fund management becomes seamless due to fast and secure deposit and withdrawal methods such as bKash, Nagad, Skrill, Neteller, bank transfers, even cryptocurrency!
OneTo be fair, what makes R777 Casino really different is its commitment to a flawless and safe gambling experience. Players can rest easy knowing they can enjoy their favorite games without worry thanks to fair play policies, responsible gambling tools and a seamless app experience. Be it a major cashout on Aviator or Crash or an odds boost on a sports wager, a platform is where you go for the best in high-octane, top-tier gaming action.
Now it’s your turn. Sign up today to receive your welcome bonus and you can begin to play! Join us today, because whether you’re here to play for play or for the next big jackpot, every second counts!